Streamlining Your Engineering Projects with Machine Learning Techniques
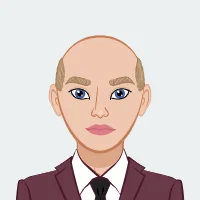
In the fast-paced world of technology and engineering, staying ahead of the curve is crucial for success. As industries continue to evolve, incorporating cutting-edge technologies becomes imperative for efficient project management. One such revolutionary technology that has made significant strides in recent years is Machine Learning (ML). In this blog post, we will explore the ways in which ML techniques can be leveraged to streamline engineering projects, enhance productivity, and achieve better results. In the relentless pursuit of success within the dynamic intersection of technology and engineering, the imperative to remain at the vanguard of innovation has never been more pronounced. Whether you're a student looking for help with your Machine Learning assignment or an industry professional eager to integrate ML into your projects, this post aims to illuminate the transformative potential of ML in the realm of engineering.
The contemporary industrial landscape is characterized by perpetual evolution, demanding the seamless integration of cutting-edge technologies to orchestrate efficient and effective project management. In this ever-changing milieu, Machine Learning (ML) has emerged as a transformative force, charting remarkable strides in recent years and reshaping the contours of engineering endeavors. This blog post embarks on a comprehensive exploration of the multifaceted ways in which ML techniques can be strategically harnessed, propelling engineering projects towards enhanced efficiency, heightened productivity, and ultimately, the attainment of superior results.
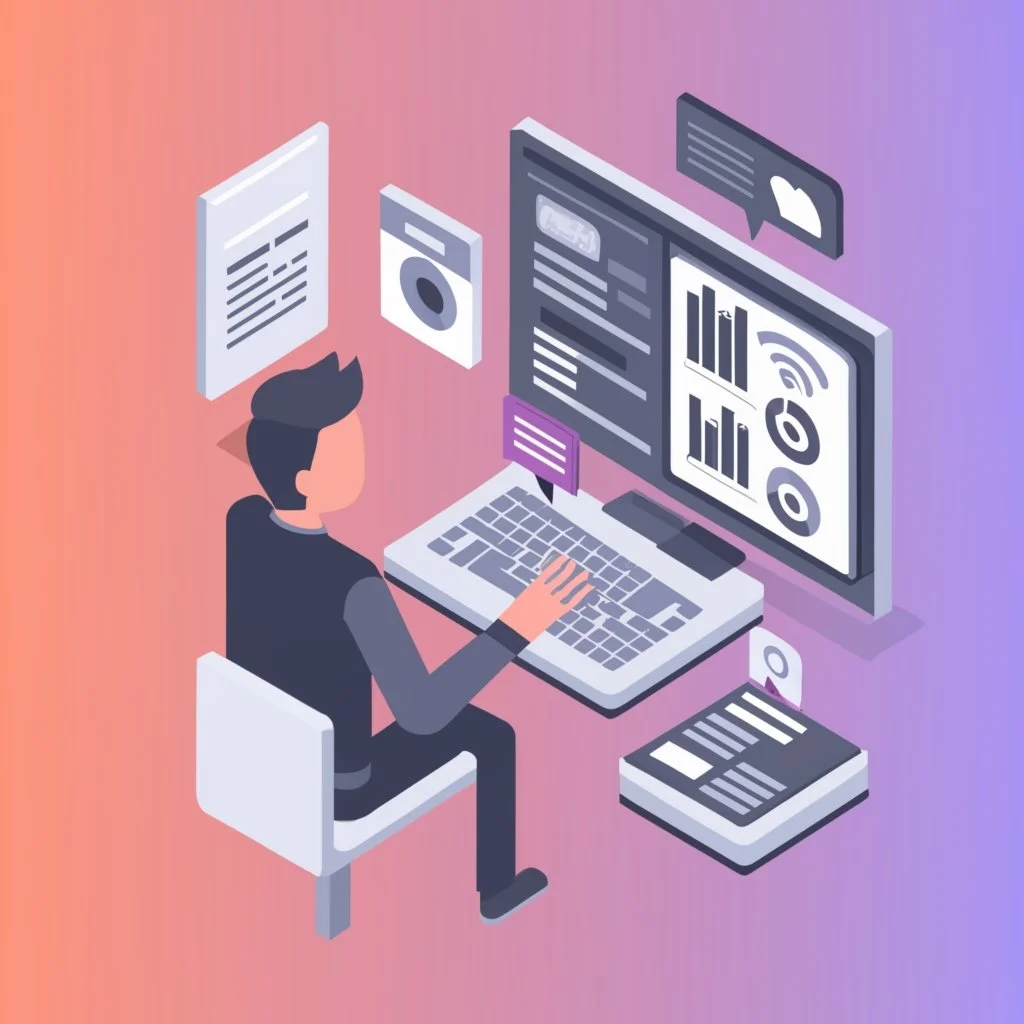
As industries undergo continuous metamorphosis, the transformative potential of ML becomes increasingly evident. At its core, ML represents the convergence of data science and artificial intelligence, enabling systems to learn from data patterns and make informed predictions or decisions. In the context of engineering projects, this amalgamation of technology offers a myriad of possibilities. To grasp the depth of its impact, we can begin by examining the role of ML in predictive modeling, a domain where historical data meets advanced algorithms to chart the course for optimized project timelines.
Predictive modeling, a linchpin of ML applications, holds the promise of revolutionizing project management. By delving into historical project data, ML algorithms can discern patterns and trends, offering insights into potential bottlenecks, resource needs, and risk factors. This not only facilitates the identification of probable project pitfalls but also empowers project managers to make informed decisions to mitigate risks, optimize timelines, and allocate resources judiciously. In essence, predictive modeling becomes a beacon of foresight, allowing engineering teams to navigate through the uncertainties of project execution with a proactive and informed approach.
Within the realm of predictive modeling, the optimization of project timelines emerges as a critical focus. Traditional project management often grapples with unforeseen delays and challenges, leading to increased costs and potential client dissatisfaction. ML-driven predictive modeling disrupts this paradigm by providing a nuanced understanding of the factors influencing project timelines. By scrutinizing historical project data, machine learning algorithms can identify critical milestones, potential roadblocks, and dependencies, facilitating the creation of realistic and achievable project timelines. The result is a paradigm shift in project planning, where foresight and precision replace reactionary measures, enabling engineering teams to adhere to schedules and deliver projects with greater efficiency.
Complementary to the optimization of project timelines, ML offers invaluable contributions to resource allocation and management—a perennial challenge in project execution. Traditional resource allocation often relies on static plans that may not adapt well to the dynamic nature of engineering projects. ML algorithms, on the other hand, can analyze historical data on resource usage, identifying patterns and fluctuations in demand. Armed with this insight, project managers can optimize resource allocation, ensuring that personnel, equipment, and materials are deployed judiciously. The outcome is a more agile and responsive approach to resource management, mitigating the risk of shortages, enhancing overall productivity, and ultimately contributing to the successful execution of engineering projects.
As industries undergo continuous evolution, the adoption of cutting-edge technologies becomes instrumental in the pursuit of efficient project management. One such technology, Machine Learning (ML), has emerged as a transformative force, making remarkable advancements in recent years. This blog post aims to unravel the diverse ways in which ML techniques can be seamlessly integrated into engineering projects, serving as a catalyst for heightened efficiency, productivity, and ultimately, superior outcomes. Against the backdrop of rapid industry evolution, ML stands out as a beacon of progress, offering unparalleled opportunities for insight and optimization. Whether through predictive modeling to refine project timelines or automated defect detection to elevate quality control standards, the impact of ML resonates across various facets of engineering endeavors. This exploration underscores the pivotal role of ML, not merely as an option but as an indispensable tool for success in the ever-shifting technological landscape. As we navigate the complexities of modern engineering, embracing the transformative potential of ML emerges as a strategic imperative, ensuring that projects not only keep pace with the times but also surpass expectations in delivering innovation and efficiency.
As we delve deeper into the transformative impact of ML, the focus shifts towards the realm of quality control—an imperative in engineering projects across diverse domains. Traditional quality control methods often entail meticulous manual inspections, a time-consuming process that may not be foolproof. ML, with its capability for automated defect detection, revolutionizes this aspect of project management. By leveraging algorithms trained on historical defect data, ML systems can automate the detection of anomalies and defects, be it in software code, manufacturing processes, or structural designs. The result is a substantial reduction in the margin for error, ensuring that deliverables meet stringent quality standards. In essence, ML-driven quality control emerges as a linchpin for engineering projects, guaranteeing not only efficiency but also the delivery of high-quality outcomes.
In the broader spectrum of performance monitoring and optimization, ML continues to assert its transformative influence. Continuous monitoring of project performance is imperative for identifying areas that require improvement and ensuring that projects stay on course. ML algorithms can analyze real-time performance data, flagging potential issues, and recommending optimizations. This extends across various engineering disciplines—whether it's optimizing code in software development or fine-tuning manufacturing processes. ML-driven insights empower engineering teams with the ability to proactively address challenges, ensuring that projects are not just executed but optimized for peak performance.
Concomitantly, ML emerges as a pivotal player in risk management and mitigation, an inherent aspect of every engineering project. By analyzing historical project data, machine learning algorithms can identify potential risks and vulnerabilities, ranging from resource shortages to external factors influencing project timelines. Armed with this foresight, project managers can implement proactive measures to mitigate risks, ensuring smoother project execution and minimizing the likelihood of costly setbacks. In this way, ML becomes a proactive ally in navigating the complexities of project management, providing a strategic advantage in risk assessment and mitigation.
The integration of Machine Learning techniques into engineering projects heralds a new era of efficiency, precision, and innovation. From the optimization of project timelines through predictive modeling to the automation of quality control processes, ML offers a comprehensive toolkit for engineering teams seeking to not only meet but exceed expectations. As industries continue their rapid evolution, embracing the transformative potential of ML becomes not just a choice but an imperative for those striving for success in the competitive landscape of modern engineering. In this era of constant innovation, ML stands as a beacon, guiding engineering projects towards a future where efficiency, quality, and success converge seamlessly.
Harnessing the Power of Predictive Modeling
Predictive modeling is a key aspect of machine learning that involves using algorithms and statistical models to analyze historical data and make predictions about future outcomes. In engineering projects, predictive modeling can be a game-changer, offering insights that enable proactive decision-making and risk mitigation.
In the dynamic realm of engineering projects, where precision and efficiency are paramount, the incorporation of cutting-edge technologies has become indispensable. Among these transformative innovations, Machine Learning (ML) stands out as a beacon of potential, offering unprecedented capabilities for streamlining and optimizing various aspects of project management. At the forefront of ML's impact is the harnessing of predictive modeling, a potent tool that transcends traditional approaches by analyzing historical data and extrapolating future trends. In the context of engineering projects, predictive modeling emerges as a strategic powerhouse, allowing teams to navigate complexities with foresight and finesse. By delving into the intricacies of project timelines, ML algorithms unveil patterns and potential bottlenecks, enabling proactive decision-making to optimize schedules and allocate resources judiciously. This paradigm shift in project planning fosters not only efficiency but also resilience, mitigating the risks of delays and enhancing overall project success. In the journey toward project excellence, the adoption of predictive modeling through machine learning serves as a linchpin, empowering engineering teams to navigate uncertainties with confidence and chart a course toward unparalleled project success.
Beyond its temporal dimensions, the impact of predictive modeling extends to resource allocation and management, addressing a perennial challenge in engineering endeavors. Through meticulous analysis of historical resource usage data, machine learning algorithms provide invaluable insights into optimal resource allocation strategies. This goes beyond mere optimization; it transforms resource management into a finely tuned orchestration, ensuring that the right resources are deployed at the right time and place. This not only prevents shortages but also cultivates an environment where resources are utilized to their fullest potential, amplifying productivity throughout the project lifecycle. As engineering projects evolve in complexity and scale, the adept utilization of predictive modeling becomes not just a tool but a strategic imperative, shaping the very fabric of project management methodologies and laying the foundation for a future where efficiency and excellence go hand in hand.
The transformative influence of predictive modeling within engineering projects extends even further, permeating the very fabric of decision-making processes and strategic planning. In the realm of project timeline optimization, machine learning algorithms become invaluable allies, offering a nuanced understanding of historical project data that transcends conventional analyses. By discerning patterns, correlations, and potential obstacles, these algorithms empower project managers to make informed decisions that resonate across the entire project lifecycle. From conceptualization to delivery, the proactive integration of predictive modeling engenders a paradigm shift in how teams approach project timelines, fostering a culture of adaptability and responsiveness. The result is not merely adherence to schedules, but the ability to navigate unforeseen challenges with agility, ensuring that engineering projects unfold seamlessly.
As the digital age ushers in an era of data-driven decision-making, predictive modeling becomes a cornerstone in deciphering the intricate tapestry of project complexities. Beyond the straightforward identification of timelines, machine learning algorithms delve into the intricacies of various project components, extracting insights that are instrumental in driving strategic initiatives. Whether it's optimizing resource allocation or anticipating potential risks, the multifaceted nature of predictive modeling positions itself as a central pillar in the arsenal of tools available to modern engineering project managers.
Resource allocation and management, often a delicate balancing act in the world of engineering, undergo a profound transformation with the infusion of predictive modeling. Machine learning algorithms scrutinize historical data with a discerning eye, offering recommendations that transcend traditional resource planning. This not only ensures that projects are adequately staffed but also mitigates the risks associated with underutilized or overburdened resources. The optimization achieved through predictive modeling has a ripple effect, permeating through the project's various facets and yielding dividends in terms of both cost-effectiveness and productivity.
In the dynamic landscape of engineering projects, where uncertainties abound and risks loom on the horizon, predictive modeling serves as a stalwart guardian, guiding teams through uncharted territories. The insights derived from historical data analysis empower teams to foresee potential pitfalls and proactively implement measures to mitigate risks. The result is not merely risk management but risk anticipation — a transformative shift from reactive crisis resolution to proactive risk avoidance. Machine learning algorithms become the sentinels, standing guard against potential disruptions and enabling engineering projects to navigate turbulent waters with poise and precision.
Beyond the temporal and resource dimensions, predictive modeling becomes a linchpin in fostering a culture of continuous improvement within engineering projects. By providing real-time feedback and insights, machine learning algorithms contribute to ongoing performance monitoring and optimization. This iterative process of refinement ensures that projects are not static entities but dynamic endeavors that evolve and adapt based on data-driven insights. Whether it's fine-tuning code in software development or optimizing manufacturing processes, the integration of predictive modeling engenders a perpetual cycle of improvement, elevating the overall performance standards of engineering projects.
The harnessing of predictive modeling within engineering projects represents a pivotal moment in the evolution of project management methodologies. As machine learning algorithms unravel the intricacies of historical data, project timelines become more than schedules—they become dynamic roadmaps, adapting to the ever-changing landscape of engineering projects. The optimization of resources goes beyond efficiency; it becomes a strategic imperative that propels projects toward success. Predictive modeling becomes a guardian against risks, an oracle that foretells potential challenges and enables teams to navigate uncertainties with finesse. In the ever-evolving landscape of engineering, the integration of predictive modeling through machine learning is not just a technological advancement; it is a paradigm shift that defines the future of project excellence.
Project Timeline Optimization
One of the primary challenges in engineering projects is managing timelines effectively. Delays can lead to increased costs and potential client dissatisfaction. Machine learning algorithms can analyze past project data, identify patterns, and predict potential bottlenecks. By incorporating these insights into project planning, teams can optimize timelines, allocate resources efficiently, and reduce the likelihood of delays.
Resource Allocation and Management
Resource allocation is a critical aspect of project management. Machine learning algorithms can analyze historical data on resource usage, identify peak demand periods, and recommend optimal resource allocation strategies. This not only helps in avoiding resource shortages but also ensures that resources are utilized effectively, maximizing productivity throughout the project lifecycle.
Enhancing Quality Control through Machine Learning
Quality control is a cornerstone of successful engineering projects. Machine learning techniques can significantly improve the accuracy and efficiency of quality control processes, leading to higher-quality deliverables. In the realm of engineering, where precision and reliability are paramount, the quality control process stands as a linchpin determining project success. Here, the fusion of traditional methodologies with cutting-edge technologies becomes imperative, and Machine Learning (ML) emerges as a powerful ally. The application of ML techniques for enhancing quality control in engineering projects represents a paradigm shift, redefining the very essence of meticulous scrutiny.
At its core, ML introduces a dynamic approach to defect detection and quality assurance, significantly altering the landscape of manual inspection. By leveraging historical data, ML algorithms exhibit a capacity to automate defect detection with unprecedented accuracy, spanning a spectrum from identifying structural vulnerabilities to pinpointing anomalies in manufacturing processes. This automated precision not only expedites the inspection process but also elevates the overall quality of deliverables. Furthermore, the real-time monitoring capabilities of ML contribute to ongoing performance optimization, allowing engineering teams to identify and rectify potential issues swiftly. The infusion of ML into the quality control fabric of engineering projects is not just an augmentation but a revolution, promising not only to streamline processes but also to elevate the industry's standard for precision and reliability.
In the intricate tapestry of engineering endeavors, the quest for perfection is a perpetual journey, and nowhere is this pursuit more critical than in the realm of quality control. As engineering projects become increasingly complex and multifaceted, the reliance on Machine Learning (ML) to fortify and finesse quality control processes becomes not merely a strategic advantage but a necessity. The traditional manual methods of defect detection and quality assurance, while meticulous, often fall short in the face of the intricate and vast datasets that characterize modern engineering projects. Machine Learning, however, presents a transformative solution, breathing life into a new era of efficiency and accuracy. By delving into the historical intricacies of project data, ML algorithms unravel patterns and intricacies that elude human observation. The beauty of this lies in the ability to automate defect detection, transcending the limitations of human bandwidth and unlocking a realm of possibilities. From scrutinizing intricate structures for vulnerabilities to discerning subtle anomalies in manufacturing processes, ML stands as a sentinel, tirelessly guarding the gates of project quality. The real magic unfolds in the expeditious nature of this automated scrutiny, accelerating the inspection process and, in turn, amplifying the overall quality of deliverables.
As ML algorithms seamlessly integrate into real-time monitoring, they become not just guardians but active partners in the pursuit of perfection. They identify emerging issues in the blink of an eye, empowering engineering teams to address concerns promptly and thereby fostering a culture of ongoing performance optimization. In this union of human expertise and machine precision, the landscape of quality control is not just evolving but undergoing a profound metamorphosis, promising not just streamlined processes but a qualitative leap in the industry's commitment to precision and reliability. The story of enhancing quality control through Machine Learning is not merely one of technological advancement; it is a narrative of an industry poised on the brink of a renaissance, where every defect detected is a step closer to perfection achieved.
In the ever-evolving saga of engineering pursuits, where intricacy meets innovation, the role of quality control takes center stage. With each project demanding a meticulous dance between precision and efficiency, the incorporation of Machine Learning (ML) into quality control processes is a transformative overture. In the face of intricate project datasets and the relentless march of technological complexity, traditional methods of defect detection find themselves at a crossroads. Herein lies the crux of ML's significance, transcending the limits of manual scrutiny. Through a deep dive into historical project intricacies, ML algorithms decode patterns that might elude human cognition.
This metamorphosis empowers the automation of defect detection, offering a dynamic solution to the challenges posed by the sheer volume and complexity of modern engineering projects. The discerning eye of ML spans from scrutinizing the most intricate structures for vulnerabilities to uncovering subtle irregularities in manufacturing processes, ushering in a new era of precision. What sets ML apart is not just its ability to expedite the inspection process, but its profound impact on the very essence of quality in deliverables. As ML algorithms seamlessly integrate into the real-time heartbeat of projects, they become vigilant custodians, proactively identifying emerging issues. This alliance between human intuition and machine precision isn't just a technological symphony; it's a revolution. It transforms quality control from a checkpoint into a dynamic process of ongoing improvement. In this narrative of integration, the story isn't just about detecting defects; it's about refining and elevating the industry's commitment to perfection. The journey of enhancing quality control through Machine Learning is not just a chapter in the history of engineering; it's a testament to the resilience of innovation, where every automated detection is a testament to progress, and every stride forward is a testament to the limitless possibilities at the intersection of human ingenuity and machine acumen.
Automated Defect Detection
Detecting defects in engineering projects is a time-consuming task that often requires meticulous manual inspection. Machine learning algorithms, trained on historical defect data, can automate the detection process. From identifying structural weaknesses to spotting anomalies in manufacturing processes, ML-powered systems can enhance the accuracy of defect detection, reducing the likelihood of errors in the final deliverables.
Performance Monitoring and Optimization
Continuous monitoring of project performance is essential for identifying areas that require improvement. Machine learning algorithms can analyze real-time performance data, flag potential issues, and recommend optimizations. Whether it's optimizing code in software development or fine-tuning manufacturing processes, ML-driven insights enable proactive decision-making to enhance overall project performance.
Risk Management and Mitigation
Every engineering project involves a degree of risk. Machine learning can contribute significantly to risk management by analyzing historical project data to identify potential risks and vulnerabilities. By understanding the factors that contribute to project failure, teams can implement proactive measures to mitigate risks, ensuring smoother project execution.
In conclusion, the integration of machine learning techniques into engineering projects has the potential to revolutionize the industry. From optimizing project timelines to enhancing quality control processes, ML-driven insights empower engineering teams to make informed decisions, ultimately leading to more successful and efficient project outcomes. As technology continues to advance, embracing machine learning is not just a choice but a necessity for those looking to stay at the forefront of the ever-evolving engineering landscape.
Conclusion
The integration of Machine Learning (ML) techniques into engineering projects emerges as a transformative force, reshaping the landscape of project management. Harnessing the power of predictive modeling allows for the optimization of project timelines and resource allocation, enabling proactive decision-making and risk mitigation. Furthermore, ML contributes to quality control by automating defect detection and facilitating real-time performance monitoring, thereby enhancing overall project performance. The technology's role in risk management is paramount, as it identifies potential pitfalls and provides insights to preemptively address challenges. As engineering projects continue to evolve, the seamless integration of ML not only streamlines processes but also fosters a culture of innovation and adaptability, positioning organizations at the forefront of success in an ever-advancing technological era. Embracing machine learning is no longer an option but a strategic imperative for those seeking to propel their engineering projects towards unprecedented efficiency and success.
Resource allocation, another cornerstone of effective project management, undergoes a profound transformation with the infusion of ML. Traditionally, resource allocation decisions relied heavily on historical data and expert intuition. However, ML algorithms, drawing on vast datasets and learning from patterns, contribute a level of precision and foresight previously unattainable. By understanding peak demand periods, optimizing workforce distribution, and accounting for variable resource needs, ML-driven insights pave the way for resource management strategies that are not only efficient but also dynamic, adapting in real-time to the evolving demands of a project.
Performance monitoring and optimization, critical components of any engineering project, undergo a renaissance with ML. Real-time analysis of project performance metrics allows for immediate identification of areas requiring attention. Whether it's code optimization in software development or fine-tuning manufacturing processes, ML-driven insights provide actionable recommendations for optimization. This not only enhances project performance but also establishes a feedback loop that facilitates continuous improvement throughout the project lifecycle. By embracing ML in performance management, engineering teams position themselves at the forefront of efficiency and innovation, adapting dynamically to the demands of an ever-changing technological landscape.
Yet, perhaps one of the most significant contributions of ML to engineering projects lies in its role in risk management and mitigation. Every project inherently carries a degree of risk, and identifying potential pitfalls is instrumental in ensuring project success. ML, with its ability to analyze vast datasets and identify patterns indicative of potential risks, empowers project managers to implement proactive measures. By understanding the factors that historically contribute to project failure, teams can take preemptive action, implementing strategies to mitigate risks before they escalate. The result is a more resilient project framework, capable of navigating uncertainties with strategic precision and minimizing the impact of unforeseen challenges.
In the grand tapestry of engineering project management, the integration of ML emerges not as a standalone enhancement but as a catalyst for cultural and procedural transformation. It fosters a culture of innovation, adaptability, and continuous improvement, where data-driven insights guide decision-making, and projects unfold as dynamic, living entities. Embracing machine learning is not merely a strategic choice; it is a progressive imperative for organizations aspiring to not only meet but exceed the expectations of the fast-paced and technology-driven engineering landscape. As we stand at the nexus of innovation, those who harness the full potential of ML stand to reshape the future of engineering projects, propelling them toward unprecedented levels of efficiency, success, and resilience.