Claim Your Offer
Unlock an amazing offer at www.programminghomeworkhelp.com with our latest promotion. Get an incredible 20% off on your second programming assignment, ensuring top-quality assistance at an affordable price. Our team of expert programmers is here to help you, making your academic journey smoother and more cost-effective. Don't miss this chance to improve your skills and save on your studies. Take advantage of our offer now and secure exceptional help for your programming assignments.
We Accept
In the ever-evolving landscape of engineering education, the ability to navigate and conquer challenging assignments has become synonymous with academic success and future professional prowess. As students grapple with the intricacies of coursework, the fusion of data mining and artificial intelligence (AI) emerges as a beacon of innovation, promising not just completion but mastery of engineering assignments. The very title, "Data Mining and AI Techniques: Completing Your Engineering Assignments," beckons us to unravel the layers of this dynamic synergy, inviting a profound exploration into the transformative capabilities that lie at the intersection of these cutting-edge technologies. Whether you require assistance with your Artificial Intelligence assignment or seek to harness the power of data mining and AI techniques in mastering engineering assignments, this blog provides valuable insights and guidance to empower you on your academic journey.
At its core, data mining serves as the backbone for extracting meaningful insights from vast and complex datasets, presenting a toolkit for students to delve into the intricacies of information gathering, pattern recognition, and informed decision-making. Simultaneously, artificial intelligence, with its capacity to emulate human intelligence, introduces a paradigm shift in problem-solving methodologies within the engineering domain. Together, these techniques offer a multidimensional approach to understanding and addressing the challenges posed by engineering assignments, propelling students into an arena where proficiency extends beyond mere task completion.
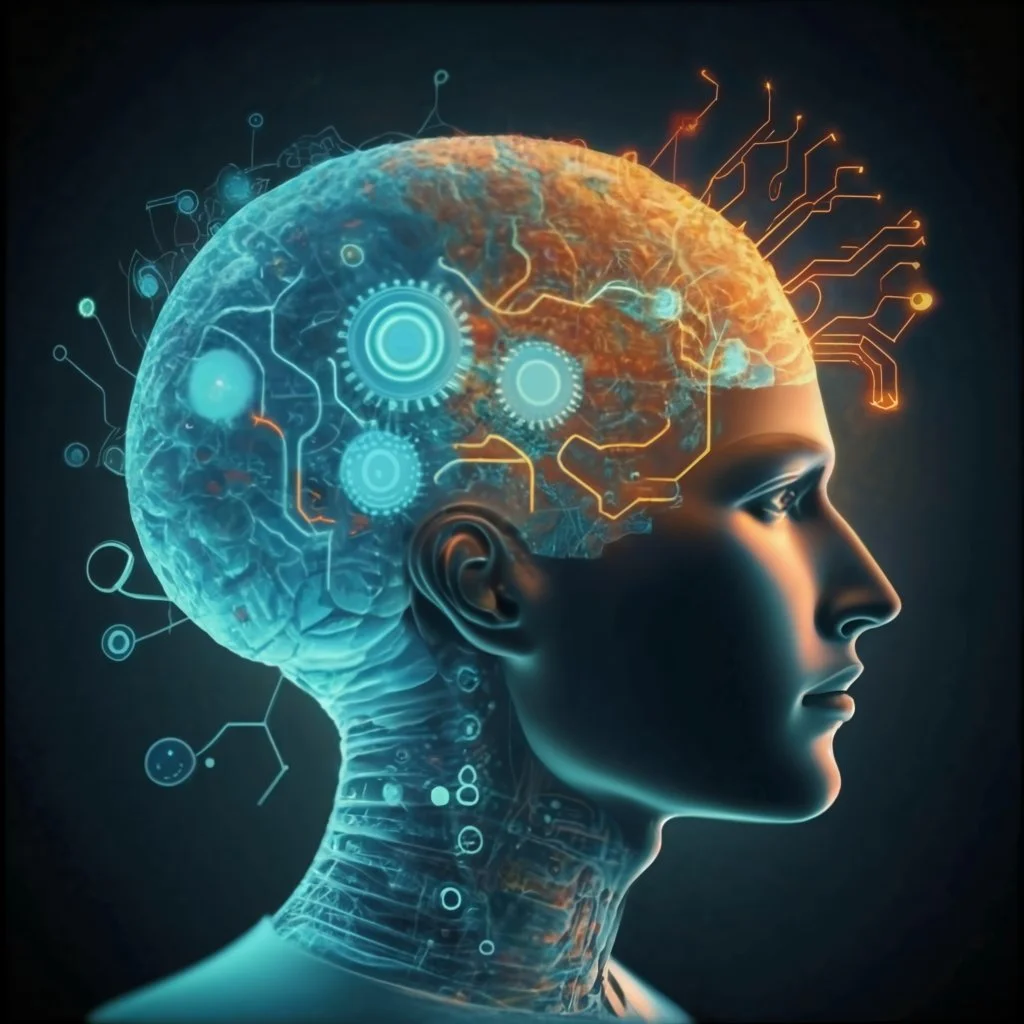
As we embark on this journey of exploration, it becomes evident that data mining and AI are not just tools for academic tasks but powerful enablers for cultivating a holistic understanding of engineering concepts. The dynamic synergy between these technologies and academic pursuits ensures that students not only meet the immediate demands of assignments but also acquire a skill set that aligns seamlessly with the evolving demands of the technological landscape.
In the chapters that follow, we will traverse the foundational landscapes of data mining, unraveling its nuances in data preprocessing, association rule mining, clustering, and classification. Simultaneously, the role of AI will be dissected, showcasing its prowess through machine learning algorithms, natural language processing, and expert systems, providing a lens through which engineering problems can be analyzed and solved with unparalleled efficiency.
The subsequent exploration of practical applications will shed light on how data mining and AI permeate diverse engineering domains, from optimizing manufacturing processes to predicting equipment failures and informing structural design. Through real-world case studies and examples, we aim to illustrate the tangible impact of integrating these technologies into the academic realm, thereby making the learning experience both engaging and relevant.
This transformative journey is not without its challenges. We will delve into the hurdles that students may encounter, from data quality issues to ethical considerations, and offer practical solutions to navigate these obstacles effectively. Recognizing that programming is often the crux of engineering assignments, we will also navigate the programming landscape, demonstrating how data mining and AI techniques can streamline coding tasks, optimize algorithms, and automate code generation.
In essence, this exploration seeks to empower students not merely with solutions to assignments but with a comprehensive understanding of how data mining and AI techniques can be harnessed as powerful allies in their academic journey. It is an ode to the integration of innovation and education, where proficiency in these transformative technologies becomes a hallmark of success in engineering assignments and a springboard for future accomplishments in the rapidly advancing technological landscape.
Understanding the Basics of Data Mining
Data mining is a crucial aspect of extracting valuable insights from large datasets. In the context of engineering assignments, it plays a pivotal role in gathering relevant information, identifying patterns, and making informed decisions. This section will delve into the fundamentals of data mining, discussing key concepts such as data preprocessing, association rule mining, clustering, and classification. By gaining a solid understanding of these basics, students can lay the foundation for tackling engineering assignments with confidence.
Understanding the basics of data mining is paramount in navigating the intricate landscape of engineering assignments. At its core, data mining is a multidisciplinary field that amalgamates techniques from statistics, machine learning, and database systems to extract meaningful patterns and knowledge from vast datasets. The process begins with data preprocessing, where raw data undergoes cleansing and transformation to ensure its quality and relevance. Once prepared, various data mining techniques come into play, including association rule mining, which identifies interesting relationships between variables; clustering, which groups similar data points together; and classification, a method that assigns labels to data based on predefined categories. These techniques collectively empower engineers to unravel hidden insights, make informed decisions, and enhance the overall understanding of complex systems.
Mastering the basics involves grasping the intricacies of these fundamental concepts, recognizing their applications, and realizing their potential in solving real-world engineering problems. As students delve into the realm of data mining, they lay the groundwork for a robust analytical skill set, one that proves invaluable in deciphering the intricacies of engineering assignments and positions them at the forefront of harnessing data for informed decision-making.
Data mining encompasses a crucial step in the data analysis pipeline known as pattern recognition, where algorithms discern regularities and trends within datasets, offering valuable insights into the underlying structures. An essential component of this process is the identification of anomalies or outliers, which might signify critical information or errors in the data. As engineers engage in data mining for their assignments, they delve into the intricacies of feature selection, choosing the most relevant attributes that contribute to the desired outcomes. Moreover, understanding the nuances of data mining involves navigating the challenges of dimensionality reduction, a technique that simplifies complex datasets by eliminating redundant or irrelevant variables, ensuring that the analysis focuses on the most pertinent information.
Data mining techniques are not confined to a singular approach; instead, they form a versatile toolkit that engineers can adapt to various scenarios. For instance, decision trees offer a transparent and interpretable framework for classification tasks, allowing engineers to follow the logical sequence of decisions made by the algorithm. Support Vector Machines (SVM), on the other hand, excel in handling complex data relationships and are particularly useful in scenarios where clear boundaries between classes are challenging to discern. By comprehending the strengths and weaknesses of each technique, engineers can strategically apply them to different aspects of their assignments, tailoring the approach to the specific challenges presented by the data at hand.
In the context of assignments, data mining serves as a bridge between raw information and actionable insights. It facilitates the identification of patterns that might otherwise remain obscured, enabling engineers to make data-driven decisions and predictions. As students dive into the intricacies of data mining, they also become adept at interpreting the results, critically evaluating the significance of discovered patterns, and ensuring the applicability of their findings to the given engineering problem. This ability to derive meaningful conclusions from data not only enhances academic performance but also nurtures a valuable skill set for future professional endeavors where data-driven decision-making is increasingly becoming a norm.
The Role of AI in Engineering Assignments
Artificial intelligence, on the other hand, empowers machines to mimic human intelligence and perform tasks that traditionally required human intervention. In the realm of engineering assignments, AI can automate processes, optimize solutions, and enhance overall efficiency. This section will explore how AI techniques, including machine learning algorithms, natural language processing, and expert systems, can be applied to solve engineering problems. Understanding the symbiotic relationship between AI and engineering assignments is crucial for students aiming to excel in their coursework.
The role of artificial intelligence (AI) in engineering assignments is transformative, revolutionizing the traditional approach to problem-solving and decision-making. AI, encompassing a spectrum of technologies that enable machines to emulate human intelligence, has emerged as a game-changer in the academic landscape. In engineering assignments, AI serves as a catalyst for innovation, offering advanced solutions to complex problems. Machine learning algorithms, a subset of AI, play a pivotal role in predictive modeling and data analysis, allowing students to make informed decisions based on patterns and trends.
Natural language processing (NLP) facilitates seamless interaction between humans and computers, aiding in the interpretation of complex engineering concepts and the development of intelligent systems. Expert systems, another facet of AI, empower students to create knowledge-based solutions, mimicking the decision-making processes of domain experts.
Through the integration of AI techniques, engineering assignments become dynamic, adaptive, and efficient, transcending the limitations of traditional problem-solving methodologies. Students engaging with AI in their assignments not only enhance their technical skills but also cultivate a forward-thinking mindset that aligns with the demands of the ever-evolving technological landscape. As AI continues to advance, its role in engineering assignments becomes increasingly indispensable, preparing students for a future where innovative problem-solving is synonymous with success in the field of engineering.
AI technologies act as intellectual companions to students, offering sophisticated tools for data analysis, pattern recognition, and decision-making. Machine learning, a cornerstone of AI, empowers engineering students to create models that learn from data, adapt to changing circumstances, and make predictions with a high degree of accuracy. Natural language processing (NLP) allows students to interact with their assignments in a more intuitive manner, enabling them to articulate complex engineering concepts and receive intelligent feedback. Expert systems, drawing on accumulated knowledge, assist students in solving intricate problems by emulating the decision-making processes of seasoned professionals. The fusion of these AI techniques not only expedites the resolution of engineering challenges but also fosters a deeper understanding of the underlying principles. As students navigate the complexities of their coursework.
AI becomes a mentor, guiding them through intricate problem spaces and offering insights that extend beyond the scope of conventional approaches. Moreover, AI-driven tools facilitate optimization, enabling students to fine-tune their solutions for efficiency and effectiveness. In essence, the role of AI in engineering assignments transcends the boundaries of routine automation; it becomes a catalyst for intellectual growth, innovation, and a more nuanced comprehension of the intricate problems inherent in the field of engineering. Embracing AI in assignments is not just a technological leap but a pedagogical evolution that equips students with the skills and mindset needed to thrive in a world where the symbiosis of human ingenuity and artificial intelligence is the hallmark of success.
Practical Applications of Data Mining and AI in Engineering
To provide a real-world perspective, this section will highlight practical applications of data mining and AI in various engineering domains. Whether it's optimizing manufacturing processes, predicting equipment failures, or analyzing large datasets for structural design, the integration of these technologies has far-reaching implications. By showcasing case studies and examples, students can grasp the tangible impact of incorporating data mining and AI techniques into their assignments, making the learning experience more engaging and relevant.
Practical Applications of Data Mining and AI in engineering extend across diverse domains, revolutionizing traditional approaches and enhancing problem-solving capabilities. In the field of manufacturing, data mining techniques enable predictive maintenance, minimizing downtime by anticipating equipment failures based on historical data patterns. AI algorithms contribute to precision control systems, optimizing production processes and ensuring higher efficiency. Structural engineering benefits from these technologies through advanced simulations and analyses, where data mining aids in identifying structural vulnerabilities, and AI assists in generating optimal designs. In healthcare, the integration of AI and data mining facilitates personalized treatment plans by analyzing patient data, predicting disease trajectories, and identifying potential risks.
Transportation systems leverage these technologies for route optimization, traffic management, and predictive maintenance of vehicles. Environmental engineering benefits from data mining in analyzing climate data and predicting environmental changes, while AI contributes to optimizing resource usage and promoting sustainable practices. In financial engineering, predictive analytics powered by data mining aids in risk assessment and fraud detection, while AI algorithms optimize investment portfolios based on market trends. The fusion of data mining and AI techniques thus spans a multitude of engineering applications, offering solutions that not only streamline processes but also pave the way for innovative approaches and advancements in diverse sectors.
The fusion of data mining and AI techniques in engineering has significantly impacted the energy sector. Data mining aids in analyzing energy consumption patterns, optimizing distribution networks, and identifying opportunities for energy conservation. AI applications in this domain include the development of smart grids that dynamically adapt to demand, predictive maintenance of power plants, and the integration of renewable energy sources for efficient energy production. In telecommunications, data mining assists in analyzing network performance, predicting potential issues, and optimizing communication protocols. AI-powered chatbots and virtual assistants enhance customer service experiences by understanding user queries and providing timely and accurate responses.
In the realm of cybersecurity, the combination of data mining and AI has become instrumental in identifying and preventing cyber threats. Data mining helps analyze vast amounts of network data to detect patterns indicative of potential security breaches, while AI algorithms enable the development of adaptive and self-learning systems that can respond to emerging threats in real-time. The synergy between these technologies has elevated the security posture of critical infrastructure and sensitive information systems.
The application of data mining and AI in the field of robotics has led to advancements in automation and autonomous systems. Robotics engineers leverage data mining to analyze sensor data, improving robot perception and decision-making capabilities. AI algorithms enable robots to adapt to dynamic environments, learn from experience, and execute tasks with precision. This integration has paved the way for the development of autonomous vehicles, drones, and robotic systems used in manufacturing, healthcare, and exploration.
The impact of data mining and AI is also evident in the field of environmental monitoring and conservation. These technologies assist in analyzing ecological data, tracking wildlife patterns, and predicting environmental changes. AI-powered drones equipped with data mining capabilities enable efficient monitoring of biodiversity, deforestation, and pollution levels, contributing to conservation efforts and sustainable resource management.
Challenges and Solutions in Implementing Data Mining and AI
While the benefits of data mining and AI in engineering assignments are immense, challenges often arise during implementation. This section will address common hurdles students may face, such as data quality issues, algorithm selection dilemmas, and ethical considerations. Moreover, it will offer practical solutions and tips to overcome these challenges, ensuring that students can navigate the complexities of integrating data mining and AI techniques into their assignments effectively. Navigating the integration of data mining and artificial intelligence (AI) into engineering assignments presents a myriad of challenges, demanding a nuanced understanding and strategic approach. A primary hurdle lies in the quality of available data, as the success of data mining and AI techniques heavily relies on the integrity and relevance of the datasets used. Inconsistent, incomplete, or biased data can skew results and hinder the effectiveness of algorithms.
Another challenge pertains to the selection of appropriate algorithms, considering the vast array available. The dilemma of choosing between supervised and unsupervised learning, decision trees or neural networks, adds complexity. Ethical considerations emerge as a critical concern, especially in applications that involve sensitive data. Striking a balance between maximizing utility and ensuring privacy and security is a delicate undertaking. Additionally, the ever-evolving nature of technology introduces the challenge of staying current with the latest advancements and industry standards. This dynamic landscape requires students to continuously update their skillsets. Nevertheless, solutions exist to address these challenges. Rigorous data preprocessing methods, including data cleaning and normalization, can enhance the quality of input data.
Strategic algorithm selection, guided by a comprehensive understanding of the problem at hand, aids in optimizing performance. Implementing ethical guidelines and robust security measures ensures responsible and secure application of data mining and AI techniques. Finally, fostering a commitment to continuous learning and professional development equips students with the resilience needed to navigate the rapidly evolving technological landscape, ensuring their proficiency in overcoming challenges and successfully integrating data mining and AI into their engineering assignments.
The implementation of data mining and AI techniques in engineering assignments is also impeded by the computational complexities associated with large datasets. The sheer volume of information often requires significant computational power, posing challenges for students who may not have access to high-performance computing resources. This limitation can hinder the scalability of certain algorithms and impede the efficient processing of extensive datasets. Additionally, the interpretability of complex models poses another challenge. As algorithms become more sophisticated, understanding the inner workings of these models becomes increasingly intricate, making it difficult for students to explain their solutions coherently.
Conclusion
In the rapidly evolving landscape of engineering education, the fusion of data mining and artificial intelligence (AI) techniques emerges as a transformative force, providing students with a formidable set of tools to navigate and excel in their assignments. The foundational understanding of data mining, encompassing key concepts such as preprocessing, association rule mining, clustering, and classification, lays the groundwork for students to unravel complex datasets and extract meaningful insights. Simultaneously, the integration of AI, with its machine learning algorithms, natural language processing, and expert systems, empowers students to automate processes, optimize solutions, and enhance the overall efficiency of their engineering assignments. The practical applications of these technologies across various engineering domains further illuminate their significance, from optimizing manufacturing processes to predicting equipment failures and analyzing large datasets for structural design. Yet, with these opportunities come challenges, ranging from data quality issues to ethical considerations, requiring students to navigate complexities with resilience. Addressing these challenges, however, opens avenues for growth and innovation.
This blog has sought to guide students through the programming landscape, showcasing how data mining and AI techniques can be valuable allies in coding tasks, optimizing algorithms, and automating code generation. As students embrace these technologies, they not only complete assignments more effectively but also acquire skills that are increasingly in demand in the dynamic field of technology. In conclusion, the marriage of data mining and AI techniques equips students not only with the ability to conquer engineering assignments but also with a profound understanding of technologies that will shape the future of their careers in this ever evolving and demanding landscape.
The tangible applications of these symbiotic technologies across diverse engineering domains underscore their transformative impact. From optimizing manufacturing processes and predicting equipment failures to analyzing expansive datasets for precise structural design, the practical implications of data mining and AI unfold as invaluable assets. Yet, this technological landscape is not devoid of challenges. The blog has shed light on the hurdles students might encounter, including data quality issues, algorithmic conundrums, and ethical considerations, urging them to approach these complexities with resilience and innovative problem-solving.
In traversing the programming landscape, this exploration has spotlighted how data mining and AI techniques serve as indispensable allies. Whether through the streamlining of coding tasks, optimization of algorithms, or the automation of code generation, students gain insights into the transformative potential of these technologies in enhancing their programming acumen. Embracing these innovations is not merely a means to assignment completion; it is an investment in a skill set that aligns seamlessly with the demands of the technology-driven future.
As this blog concludes, it emphasizes that the integration of data mining and AI techniques is not merely a strategy for academic success but a pathway to proficiency in technologies that will define the careers of students in an era marked by technological dynamism. Beyond the immediate challenges of assignments, students are cultivating skills that are not only pertinent but increasingly essential in a professional landscape that values adaptability, innovation, and a deep understanding of cutting-edge technologies. In uniting the realms of data mining, AI, and engineering assignments, this fusion offers students a transformative journey towards mastery in both academic pursuits and the broader landscape of technological innovation and application.