Claim Your Offer
Unlock an amazing offer at www.programminghomeworkhelp.com with our latest promotion. Get an incredible 10% off on your all programming assignment, ensuring top-quality assistance at an affordable price. Our team of expert programmers is here to help you, making your academic journey smoother and more cost-effective. Don't miss this chance to improve your skills and save on your studies. Take advantage of our offer now and secure exceptional help for your programming assignments.
We Accept
- Understanding Machine Learning in Education
- Personalized Learning Paths
- Predictive Analytics for Student Performance
- Intelligent Tutoring Systems
- Enhancing Curriculum Design
- Curriculum Optimization through Data Analysis
- Adaptive Learning Materials
- Facilitating Research and Development
- Data-Driven Educational Research
- Predictive Modeling for Educational Trends
- Challenges and Considerations
- Ethical Considerations in Machine Learning Education
- Faculty Training and Development
- Conclusion
In the dynamic realm of technology, the integration of machine learning has emerged as an indispensable force, permeating various fields with transformative effects. Particularly noteworthy is its profound impact on engineering education, where machine learning is spearheading significant advancements. This blog embarks on an exploration of the practical applications of machine learning in the context of engineering education, unraveling the ways in which it is reshaping the learning experience for students. As technology continues its relentless evolution, the intersection with education becomes increasingly crucial, and machine learning stands as a pivotal catalyst in this convergence. Within the realm of engineering education, the infusion of machine learning promises a paradigm shift, offering tailored solutions and innovative approaches that adapt to the diverse learning needs of students. Beyond its technical prowess, this integration raises important questions about ethical considerations and underscores the need for comprehensive faculty training. Thus, this blog not only delves into the transformative potential of machine learning in engineering education but also navigates the challenges and opportunities that accompany this technological frontier, painting a comprehensive portrait of the evolving landscape where academia and artificial intelligence intersect. If you need assistance to complete your Machine Learning assignment, consider exploring the dynamic integration of this technology in the context of engineering education, understanding its transformative potential, and addressing the ethical considerations and challenges associated with its implementation.
Understanding Machine Learning in Education
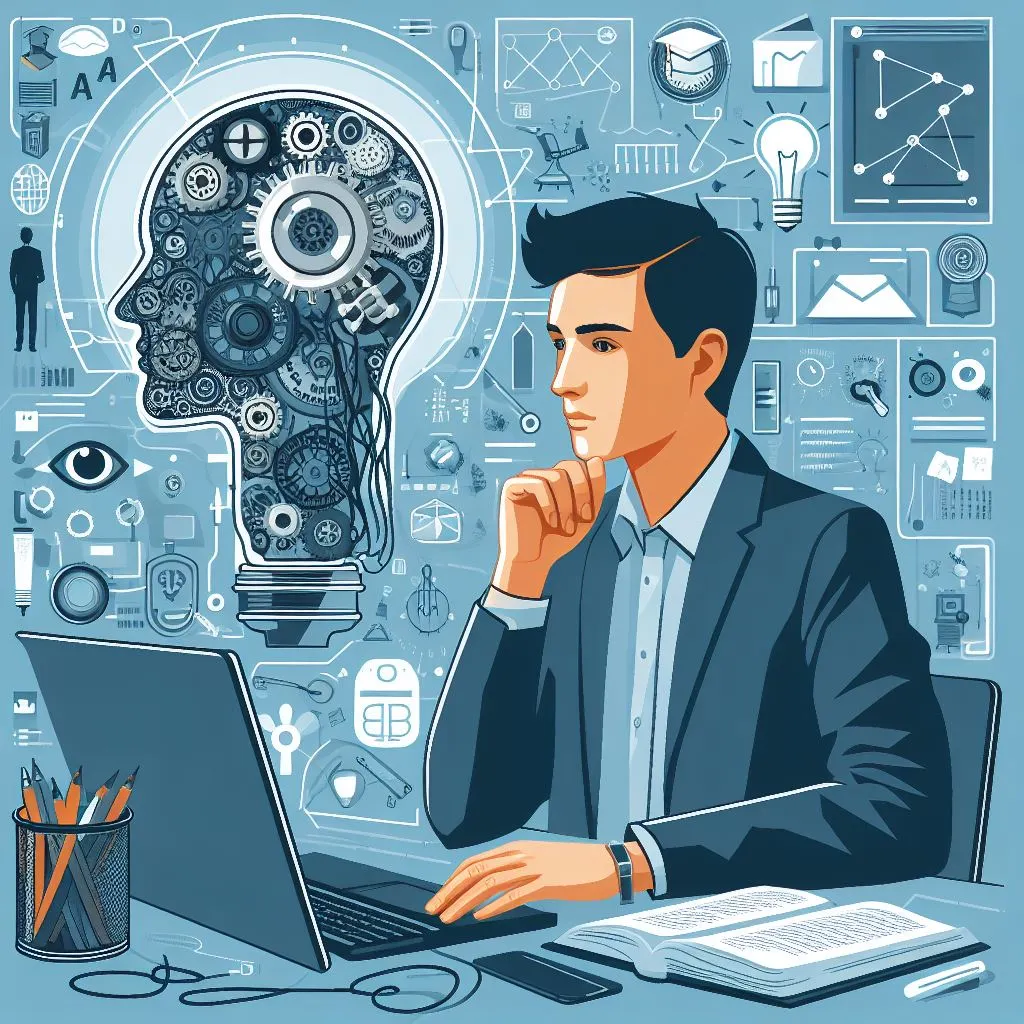
Before exploring the practical applications, it is crucial to grasp the essence of machine learning in the educational context. Machine learning, a subset of artificial intelligence, operates through algorithms and statistical models, enabling systems to execute tasks without explicit programming. In education, machine learning serves as a powerful tool for analyzing data, making predictions, and refining the overall learning process. By delving into the fundamental principles of machine learning, we lay the groundwork for comprehending its transformative applications in engineering education. This understanding illuminates the potential of machine learning to adapt, personalize, and optimize educational experiences, setting the stage for a more nuanced exploration of its impact on shaping the future of engineering learning environments.
Personalized Learning Paths
In the realm of engineering education, a transformative application of machine learning lies in the creation of personalized learning paths for students. Departing from traditional one-size-fits-all approaches, machine learning algorithms delve into individual student performance metrics, preferences, and learning styles. This granular analysis allows for the tailoring of educational content to meet the specific needs of each student. The result is a dynamic and personalized guidance system that goes beyond conventional teaching methods, fostering an educational experience that is not only more effective but also attuned to the unique strengths and challenges of each learner. This exploration of personalized learning paths highlights the potential of machine learning to revolutionize traditional education paradigms and elevate the effectiveness of engineering education to new heights.
Predictive Analytics for Student Performance
Machine learning algorithms delve into multifaceted data points, including previous academic records, engagement with course materials, and online participation, to craft a comprehensive predictive analytics model for student performance. This intricate analysis equips educators with a nuanced understanding of individual learning trajectories, enabling proactive identification of students at risk of falling behind. Beyond the initial assessment, these predictive insights form the foundation for targeted interventions, facilitating a personalized approach to assistance and support. The incorporation of machine learning in this realm empowers educational institutions to not only reactively address challenges but also proactively implement early intervention strategies. This holistic approach fosters an environment where the emphasis is not solely on addressing academic shortcomings but on nurturing individual potentials, ultimately contributing to a more holistic and tailored educational experience.
Intelligent Tutoring Systems
In the realm of engineering education, the integration of machine learning breathes life into Intelligent Tutoring Systems (ITS), marking a revolution in instructional methodologies. These systems, driven by machine learning algorithms, transcend conventional teaching approaches by adapting to the unique needs of individual students. In a dynamic scenario, an intelligent tutoring system within an engineering course goes beyond mere assessment, actively engaging with a student's problem-solving approach. By identifying areas of struggle, these systems offer real-time, customized feedback and hints, providing additional resources strategically tailored to enhance comprehension. This not only augments the quality of understanding but also propels a shift towards a self-paced learning environment. The symbiosis of machine learning and intelligent tutoring systems represents a seismic shift in educational paradigms, creating an ecosystem where technological innovation is harnessed to amplify the efficacy of individualized instruction in engineering education.
Enhancing Curriculum Design
Within the landscape of engineering education, machine learning emerges as a pivotal force in reshaping the curriculum design process. Its role is integral to maintaining the relevance and dynamism of educational content. Machine learning algorithms, through the analysis of industry trends, job market demands, and emerging technologies, provide educators with insights crucial for updating and refining course materials. This proactive approach ensures that engineering students are equipped with skills and knowledge aligned with the demands of an ever-evolving technological landscape. The marriage of machine learning and curriculum design presents a forward-thinking strategy, allowing educational institutions to stay agile and responsive to industry shifts, ultimately preparing students for success in a rapidly changing professional landscape.
Curriculum Optimization through Data Analysis
The integration of machine learning in curriculum development transcends traditional approaches by harnessing the power of large datasets. Machine learning algorithms meticulously sift through vast amounts of data to discern patterns and trends in the dynamic landscape of skills demanded by the industry. This data-driven methodology becomes a guiding beacon for educators, facilitating the optimization of curricula. By seamlessly integrating emerging technologies, cutting-edge tools, and innovative methodologies, educators can ensure that engineering students graduate with a skill set finely attuned to current industry requirements. The continuous alignment of educational content with industry demands not only enhances the educational experience but also significantly boosts the employability of engineering graduates, as they step into the professional realm equipped with the latest skills and knowledge sought by employers.
Adaptive Learning Materials
In the era of machine learning, the creation of adaptive learning materials marks a groundbreaking shift in instructional design. Machine learning facilitates the dynamic evolution of learning materials based on individual student interactions. For instance, an engineering textbook infused with adaptive learning features becomes a dynamic companion to students. It can intelligently adjust difficulty levels, providing additional examples, and offering multimedia resources tailored to individual student performance. This adaptive learning approach ensures that students receive targeted support precisely when needed, fostering a deeper understanding of complex engineering concepts. As machine learning augments the adaptability of learning materials, it not only tailors the educational experience to individual needs but also cultivates a learning environment that is responsive, engaging, and adept at addressing the diverse learning styles within the realm of engineering education.
Facilitating Research and Development
Beyond its impact on the learning experience, machine learning stands as a significant contributor to research and development within the field of engineering education. The integration of machine learning tools empowers researchers to analyze vast datasets, uncovering intricate patterns and correlations that inform a nuanced understanding of student behavior and learning outcomes. This data-driven approach not only enhances the depth of educational research but also propels the field forward by fostering innovation in pedagogical strategies. The marriage of machine learning and educational research presents an opportunity to glean valuable insights that can shape the future of engineering education, offering a pathway to more effective teaching methodologies and improved learning outcomes for students. As machine learning continues to evolve, its role in facilitating research and development within the educational domain is poised to play an increasingly pivotal role in shaping the trajectory of engineering education.
Data-Driven Educational Research
The marriage of machine learning tools and educational research opens up a vast frontier for exploration and understanding. These tools empower researchers to sift through massive datasets, unveiling intricate trends, correlations, and insights within the realm of education. This data-driven methodology brings a level of nuance to educational research, enabling a more profound understanding of student behavior, learning outcomes, and the efficacy of various teaching methodologies. Armed with these insights, researchers can not only propose innovative pedagogical approaches but also actively contribute to the continual enhancement of engineering education. Machine learning, in this context, becomes a catalyst for a research landscape that is dynamic, responsive, and capable of uncovering nuanced patterns that drive the evolution of educational practices within the field of engineering.
Predictive Modeling for Educational Trends
Machine learning takes on the role of a visionary guide in educational institutions through predictive modeling, an influential tool in anticipating future trends in engineering education. By delving into historical data and dissecting industry developments, institutions gain the foresight needed to make informed decisions about resource allocation, faculty training, and infrastructure development. This forward-looking approach ensures that engineering programs don't merely keep pace with but remain at the forefront of technological advancements. As machine learning illuminates the path ahead, educational institutions can strategically position themselves to navigate the ever-changing landscape, staying attuned to emerging trends and proactively shaping the future of engineering education to align seamlessly with the demands of an evolving technological landscape.
Challenges and Considerations
As the integration of machine learning unfolds in engineering education, a spectrum of benefits emerges, but concurrently, it prompts a recognition of inherent challenges and considerations. Acknowledging the multifaceted impact of machine learning, this section delves into the potential obstacles and ethical considerations that accompany its implementation in educational settings. From issues of bias in algorithms to concerns about data privacy, an exploration of challenges is imperative for cultivating a nuanced understanding of the responsible and ethical use of machine learning in engineering education. By dissecting these challenges, educators, policymakers, and stakeholders can work collaboratively to establish guidelines, frameworks, and best practices that ensure the ethical deployment of machine learning technologies and contribute to the creation of an inclusive and equitable learning environment.
Ethical Considerations in Machine Learning Education
Amid the escalating integration of machine learning into education, the imperative of prioritizing ethical considerations looms large. The pervasive use of machine learning systems demands heightened attention to issues such as algorithmic bias, data privacy, and the transparency of decision-making processes. In the educational domain, where the impact on individuals is profound, educators and policymakers shoulder the responsibility of collaborative efforts. Establishing robust ethical guidelines and frameworks becomes paramount, ensuring that machine learning applications uphold principles of fairness and accountability. As machine learning infiltrates educational settings, this exploration of ethical considerations stands as a call to action, prompting a collective commitment to navigate the ethical challenges and pitfalls inherent in the intersection of technology and education.
Faculty Training and Development
The seamless integration of machine learning into engineering education hinges on the preparedness of faculty members to harness these innovative tools effectively. Recognizing this imperative, institutions must invest in comprehensive professional development programs that equip educators with the requisite skills and knowledge. These programs go beyond mere introduction, fostering a culture of continuous learning that ensures faculty members stay abreast of advancements in machine learning. By nurturing a cadre of educators adept at integrating cutting-edge technologies into their teaching practices, educational institutions position themselves as incubators of innovation. The commitment to faculty training becomes a cornerstone in the transformative journey towards a technologically enriched educational landscape, where faculty members are not just instructors but catalysts for the seamless fusion of machine learning and effective pedagogy.
Conclusion
In conclusion, the infusion of machine learning into engineering education marks a profound transformation in the realms of both learning and research. The applications span from the customization of learning paths to the strategic design of curricula driven by data insights, collectively enhancing the educational journey for students and educators alike. As the landscape of technology evolves, it becomes increasingly imperative for educational institutions to conscientiously adopt and integrate these advancements. Responsible implementation entails addressing ethical considerations that may arise in the use of machine learning, ensuring fairness and transparency in its applications. Moreover, prioritizing faculty training is essential to empower educators with the requisite skills to effectively leverage these technological tools in the classroom. In navigating these considerations, educational institutions not only stay abreast of technological innovations but also contribute to shaping a future where engineering education is not merely adaptive but also positioned at the vanguard of technological innovation, preparing students for the challenges and opportunities of a rapidly advancing world.